Introduction
Gartner predicts that chatbots will become the primary support channel for a quarter of all organizations by 2027.
That’s only a few years away.
Maybe you’re considering jumping on the chatbot train and you’re exploring the best generative AI platforms. Or maybe you have a chatbot in place already and you’re not sure if it’s worth the money.
Whatever your situation, you probably know that simply having a chatbot on your website isn’t enough. Just like any other tool or system, you need to continuously manage and improve its performance to get the best bang for your buck.
In this article, we’ll show you the critical key performance indicators (KPIs) you should be monitoring to ensure your bot’s working well, to maximize your chatbot ROI, and to deliver the best support experience to your customers.
Why do you need to track bot performance?
Imagine you want to improve your typing skills. You take typing classes and invest time in developing this skill.
But how do you know if the classes are effective?
You need to identify key performance indicators, like how many words you can type per minute and how accurate your typing skills are.
The same principle applies to your chatbot’s performance (or any other tool, really). Ideally, you’d have perfect clarity on how you’ll measure the success of your conversational AI chatbot rollout long before investing time and money into implementing a new platform.
Customers tend to prefer self-service solutions (when they work), but basing a business case for your chatbot on a gut feeling or generic statistics probably won’t convince your CFO.
When it comes to a customer support chatbot, you need to consider how you’ill track:
- Whether customers are taking advantage of your chatbot offering.
- If the chatbot provides accurate responses.
- Whether it has a positive impact on customer experience.
- Whether it’s worth the investment.
Adding a new tool to your support tech stack is an investment — one you hope will pay off big time in time and money savings and in customer loyalty and happiness.
You can’t get clarity on if that’s actually happening until you have clear performance data that you can understand and use to optimize future performance.
So, where do you start?
Key performance indicators to track for your chatbot
With the multitude of chatbot tools on offer today — each with their own reporting and analytics — setting the right targets can feel overwhelming.
To simplify this task, we’ve picked the six most important chatbot KPIs for you below. These metrics will help you understand your chatbot’s performance, while also fitting naturally into your bigger picture customer service KPIs.
1. Deflection rate
Deflection rate indicates the percentage of customers who did not require human assistance after interacting with your chatbot.
Deflection rate basically shows you the share of interactions your customer service team avoided due to your bot’s intervention. While it’s easy to frame this KPI in a negative way — “Why don’t you want to talk to your customers?” — deflection rate is actually a great measure of how well your bot is helping your support team by taking routing inquiries off their plate.
To calculate your deflection rate, divide the number of tickets resolved by the chatbot without human intervention by the total number of support requests over a given period. Then, multiply the result by 100%.
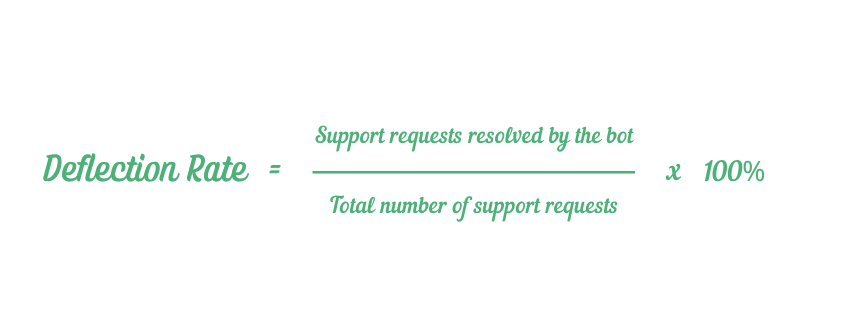
For example, if you receive 100 tickets in a month and 37 of them are deflected by your chatbot without requiring any human assistance, your deflection rate would be 37%.
Assuming customers stop the interaction because they get satisfying resolutions from the bot, deflection rate allows you to measure its effectiveness. A higher deflection rate can signal a more efficient chatbot workflow that can address various requests.
Deflection rate can vary widely across industries and products, but the recent improvements in generative AI means that bots are getting better and better at accurately resolving customer issues. Some tools — like Ultimate.ai — say they consistently deliver a deflection rate of 60% or more.
If your bot deflection rate is low, you can improve it by reviewing the tickets that required human intervention and training your bot to better handle those scenarios (and others) over time.
However, it’s important to remember that a high deflection rate doesn’t necessarily mean all customer issues were resolved, which brings us to the next crucial metric.
2. Resolution rate
Unlike deflection rate, resolution rate takes the customer perspective into account. It indicates the percentage of customers who confirmed that their issue was resolved after interacting with your bot.
In other words, the resolution rate reflects your bot’s ability to actually solve customers’ problems effectively.
To calculate your chatbot’s resolution rate, divide the number of customer confirmed support requests resolved via your bot by the total number of support requests received. Then, multiply the result by 100%.
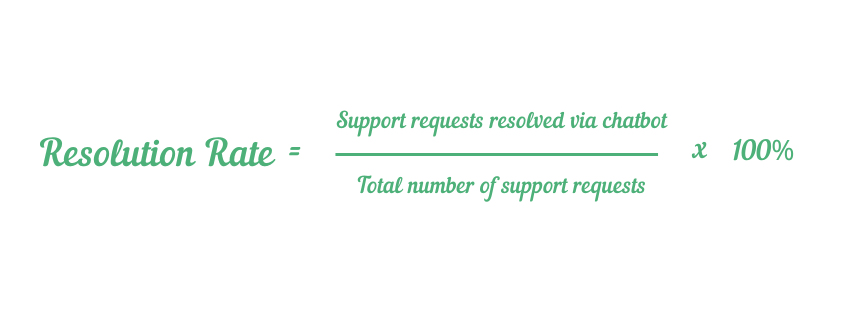
Let’s revisit our previous example. If your chatbot deflected 37 tickets, but only 20 customers confirmed that their issues were resolved, the deflection rate would still be 37%, but the resolution rate would be lower (20%).
The higher your resolution rate, the better. In this case, there are no exceptions – your goal should always be to close the loop and resolve your customers’ issues, not just deflect them.
You can improve your chatbot’s resolution rate by analyzing tickets where your chatbot couldn’t provide correct responses.
If you’re using a conversational AI bot, continue to train the AI model based on your findings.
If your team relies on a rule-based bot, adjusting your workflows to include more details in the bot’s answers or to add missing options to the decision tree can improve the accuracy and effectiveness of your bot flow.
Chatbot resolution rate also provides a clearer picture of customer satisfaction with the chatbot’s assistance. Rather than assuming a customer’s problem was fixed (like deflection rate does), resolution rate grounds things in reality.
3. Customer satisfaction (CSAT) score
CSAT score measures the level of customer satisfaction following a specific support interaction – such as an interaction with your chatbot.
CSAT surveys have been around for ages and are often used to assess the experience a human support agent is creating. Typically, customers are prompted to complete a satisfaction survey immediately after the interaction, rating their experience on a scale from 1 to 5.
Your CSAT score is usually calculated as the percentage of satisfied customers who replied to the survey with 4 or 5 ratings.

While this method is common, some organizations instead opt to use the average score for their CSAT. If you use the average approach, you’ll assign a numerical score to each rating (e.g. a 4 out of 5 is 80%, a 5 out of 5 is 100%) and then calculate the average score across all responses.
The choice of how to calculate it is yours — just ensure you’re measuring CSAT in a consistent manner over time so that you can understand if satisfaction is trending up or down.
In competitive industries like SaaS and e-commerce, the CSAT benchmark hovers around 80%.
Adding a field for open-ended feedback to your CSAT survey can also offer additional insights into the reasons behind low and high scores.
Tracking CSAT ratings separately for conversations with and without bot involvement will help you understand the impact your chatbot has on the customer experience:
- Are customers consistently indicating they’re satisfied after a bot interaction?
- How do your bot scores compare to the CSAT scores for your human agents?
- What kind trends stand out in the open-ended feedback about each?
To improve your bot’s CSAT score, review the bot’s conversations and customer feedback to identify commonalities among tickets with low and high ratings. You might find themes or issue types where customers tend to have a better experience if they speak to a human agent right away. On the other hand, you might identify topics where your chatbot consistently receives high ratings — areas you can double down on.
Customers are usually happy to get quick responses from a bot when they reach out with simple questions or requests. More complicated issues where your team has to review backend logs or account billing history, may not result in an ideal experience if routed to a bot.
4. Chatbot drop-off rate
Lengthy chatbot interactions can be exhausting for customers. If too many steps are required to resolve a query, visitors may disengage before reaching a resolution. To evaluate the effectiveness of your chatbot’s dialogue flow and identify any issues causing friction, it’s crucial to monitor the drop-off rates.
The drop-off rate measures the percentage of users who left a chatbot conversation before reaching a solution (or asking to connect with a human)
To be clear, this is different from deflection rate. Your drop-off rate only looks at customers who drop off mid-flow or mid-conversation. Deflection rate includes those customers, but it also includes customers who’ve gotten an answer or completed a flow.
You can calculate your chatbot drop-off rate by dividing the number of users who fail to complete a specific flow by the number of those who started it, and then multiplying by 100%.
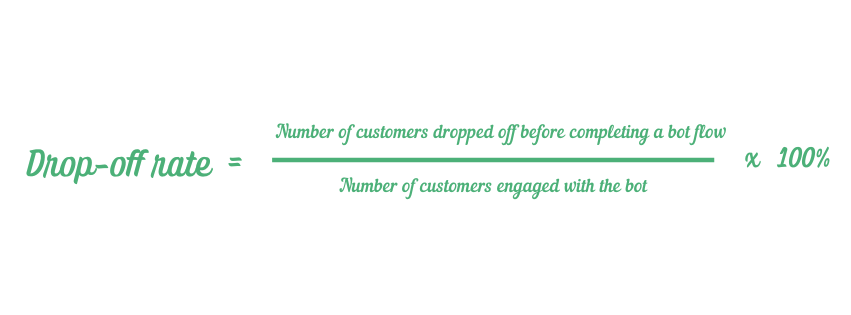
Monitoring where customers leave the flow helps identify any friction and ensures customers get the help they need when engaging with the chatbot. This is especially helpful for rule-based bots, where you can adjust specific branches with higher drop-off rates.
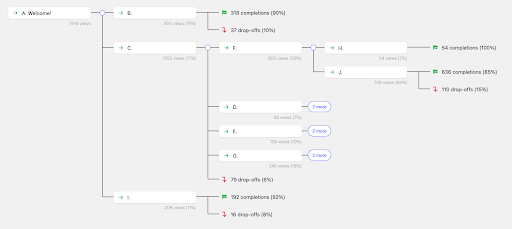
Most chatbot tools can show the drop-off rate for each step of the flow, allowing you to pinpoint weak answers and make targeted improvements.
5. Quality assurance (QA) score
Quality assurance programs are powerful tools for driving continuous service improvements and boosting customer loyalty. QA programs involve systematically reviewing support cases against predefined criteria, such as first contact resolution, tone of voice, grammar, and the efficiency of solutions provided.
Your QA scorecard will contain a number of criteria, such as “provide an accurate resolution.” Each criteria will have its own score, and, if certain items are more important than others, you might also weight items differently.
The QA score is calculated based on the percentage of met criteria or the sum of all scores a specific interaction received during the QA review.
For example, if an agent achieves everything on the below scorecard perfectly, they’ll receive a QA score of 100:
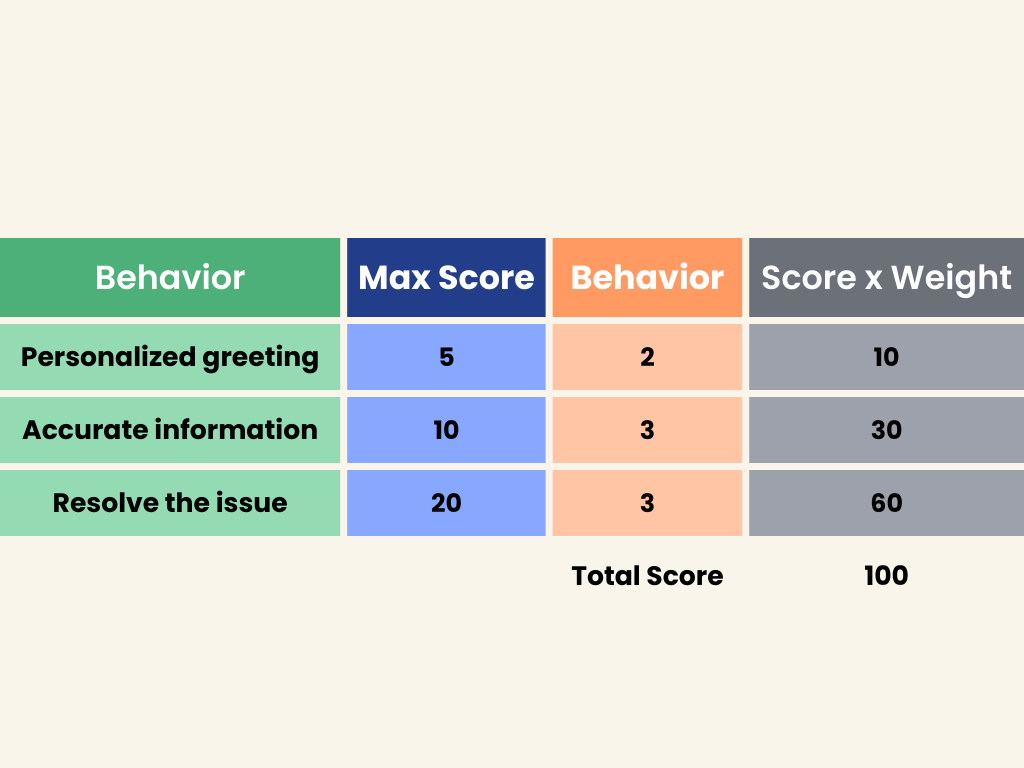
If the agent failed to understand the issue and therefore didn’t resolve it, they might get a “0” for that behavior, which means their QA score would drop down to a total of 40.
Customer service teams have used QA programs and QA scores for years, but these programs frequently aren’t extended to include chatbot conversations. While working with clients to create, improve, and implement AI and technology solutions, we’ve found that there’s often a ton of value in assessing your chatbot the same way you’d assess a human team member.
In many cases, you can actually run AI-handled conversations through the same QA scorecard you use for your human agents, which gives you a really clear baseline for understanding how bot interactions are different.
You can also use Voice of Customer (VoC) tools leveraging AI for pattern recognition, predictive analytics, and sentiment analysis. These tools can automate the QA process and assess the quality of 100% of your support interactions, giving you lots of data to compare customer sentiment from bot interactions versus human interactions.
Lastly, let’s discuss cost savings.
While this isn’t a performance metric in the same way that deflection rate or CSAT are, tracking and understanding the cost savings your bot brings is a vital part of your overall chatbot success.
Implementing any tool or process should yield a measurable return on investment (ROI). To understand the cost savings and ROI of your chatbot, you need to understand the cost of an average support conversation (often called cost per ticket) and the number of tickets handled by the bot.
By handling frequently asked questions and high volume, repetitive queries, your bot allows human agents to focus on more complex issues, leading to significant cost savings.
Put another way, a good chatbot should enable you to get the same — or more — work done with a smaller team of human agents.
To calculate the amount of money saved with bot implementation, multiply the number of tickets resolved via the bot by the average cost per ticket. If you’re paying for the chatbot tool separately and it’s not included in your general helpdesk subscription cost, subtract the cost of the chatbot service from the final result.
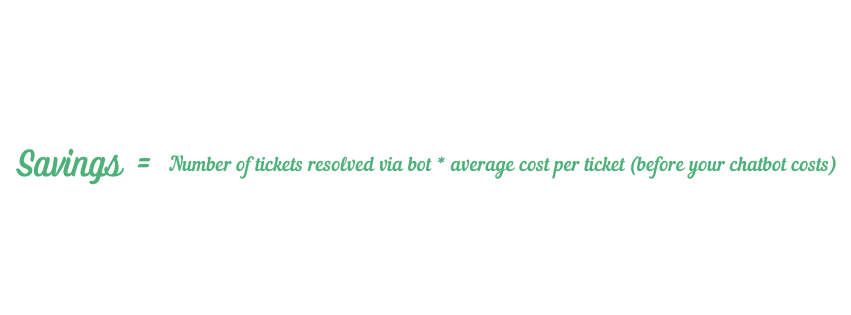
It’s important to subtract out your chatbot costs in this calculation, because you’re trying to understand what the costs would have looked like if your chatbot hadn’t been in place.
These savings can be redistributed into hiring more skilled employees, increasing salaries for existing agents, or implementing other initiatives to enhance customer experience, such as internal process automation, robust QA programs, and knowledge base software.
The exact amount saved depends on the volume of deflected tickets and the average cost of support interaction in your context. For larger organizations, the savings quickly stack up.
Beyond numbers
Tracking the right chatbot KPIs is crucial to ensuring that your efforts and systems are driving the outcomes you’re hoping for, like long-term customer satisfaction and loyalty.
At Peak Support, we live and breathe metrics like this, because we build and run effective support operations for companies of all sizes. If you’re ready to learn more, check out our Complete Guide to Customer Service KPIs with benchmark data you can use to see how your company stacks up against others.
And if you need assistance setting up your customer support tools or managing your support team, you can book a free consultation with our team today.